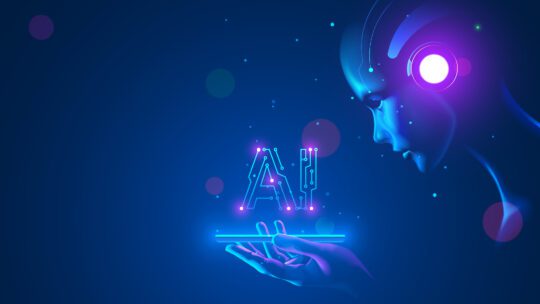
Increased awareness around and access to generative AI brings equal parts anxiety and excitement. But while there are certainly ethical issues to contend with, there are also significant advantages, including increased dexterity and capacity that comes with adding AI-powered tools to your work.
Where We Are Today
Communications analytics experts are exploring the creativity and freedom that comes with AI across analytics and primary research. The real advantages of AI integration in communications research and analytics—the incredible evolution that AI has ignited—is no longer the stuff of sci-fi fantasies. It’s what we’ve already been doing—just bigger, faster and smarter than ever before.
Bring on the Automation
The integration of AI into the existing work of communications analysts makes it easier than ever before to pull data directly from any number of different data sources, including business and media intelligence tools, in addition to first-party data sources such as sales, traffic and customer surveys. We are regularly creating data visualization treatments, including dashboards, that provide a much more holistic view of communications and marketing than was previously feasible.
Likewise, we are automating the first pass at insights using AI. (It is worth noting that we are a long way from relying on AI to develop brand-critical insights unsupervised, but it already helps us speed up that process and focus on the more complex insights and implications.)
What Communicators Can Do Now
The PESO model (Paid, Earned, Shared, Owned) still stands, but the ability to develop comprehensive and holistic reporting means that teams need to adapt. Expertise in paid, social and any other media remains essential. We need impactful content to break through the noise.
We can measure the impact of a campaign by channel and across channels, examining the impact of each component on the others. We still evaluate progress for each type, but we can quickly examine the interactions for a more fluid assessment. This capability brings a whole new meaning to holistic measurement and evaluation.
The Future is Customized
Access to many different data sources means the reporting we build is entirely customizable. We’ve always strived to create customized reporting that clearly identifies significant effects in the data and underscores what, precisely, impacts clients’ strategy and tactics. Now, our work is informed by more data and more sources of data, increasing the value of our analyses. We are analyzing proprietary first-party data alongside media and business intelligence, uncovering critical relationships and interactions.
We are measuring the value of PR with greater accuracy and meaning than we ever have before, by moving past correlations and truly linking communication outcomes to business—and vice versa. Previously, we would identify meaningful insights in discrete data sources and then qualitatively cobble them together with other data, inferring correlations and relationships. Today, AI has democratized access to, and analyses between, multiple data sources.
The Rise of First-Party Data
The flexibility and customization of analyses and reporting emphasizes the increasing value of first-party data. Integrating multiple data sources in unique ways is empowering, but integrating those sources with your own relevant data puts you in the drivers’ seat.
The more data your organization consistently collects on itself, the greater the potential insights you can develop to drive smart decision-making. First party data is more important than ever before and only likely to increase in value. AI minimizes previous barriers, including budget and bandwidth, that prevented smaller organizations from understanding their own performance. AI has significantly democratized access to the analyses providing key insights into business performance.
Connecting the Dots
It is no secret that PR has always struggled to address questions related to ROI. Indeed, it’s hard to put a price on communications, because there isn’t a direct, 1:1 relationship with sales. And frequently, a bigger issue than an indirect relationship is that performance data is housed in a different place, owned by a different group than those leading and measuring communications efforts.
AI facilitates easy integration, minimizing barriers to analysis between communications data and performance data. But we still need to thoughtfully evaluate what that relationship does and should look like. In my view, that it isn’t a 1:1 ratio; a strong reputation isn’t something you purchase once and move on.
That said, we can clearly and consistently illustrate the impact of communications on actual performance, free of models, assumptions and presumptions. AI makes it possible, sometimes even easy—and first-party data makes it invaluable.
Feel the Need for Speed
Perhaps less exciting—but no less important—is the fact that AI accelerates our work. Repetitive, time-consuming tasks previously farmed out to interns and junior staff can now be trained within an AI model. We can train models to apply an evolving sense of judgement and decision-making aptitude, too. It minimizes the time spent manually extracting data. [But note: AI does not eliminate training time. In fact, it can take just as long to train a model and spot check the results as it would a team of analysts. AI can only be as strong as the model is well fit for a specific purpose. We are a long way from AI executing anything meaningful without significant human supervision.]
AI scales our ability to conduct multiple analyses on multiple data sets in a micro-fraction of the time it would take an individual or team to do the same. This drives flexibility. When we can investigate data unconcerned about time and with little additional cost, we can spend more time finding meaningful relationships within the data.
Concerns Over Inaccuracies
The democratizing impact of AI plays out in the following ways: access to advanced analytical capabilities, limited cost of automated measurement dashboards and expedient analysis of multiple data sources.
But the concern over bias and accuracy has rightfully instigated serious conversation—because accuracy is at stake. AI-developed analyses are subject to the same challenges as human generated analyses. Data quality, availability and completeness all impact quality. And the model may or may not be trained to flag issues with incorrect, suspect or missing data.
Additionally, models are man-made—and man makes mistakes. A model built incorrectly will certainly create incorrect outputs. And again, that model may not be trained to flag inaccuracies or data conflicts, which will likely increase the time before a problem is caught and resolved.
Finally, I would posit most models are worse at recognizing false or faulty information or data than their human counterparts. Unless specially trained, AI models do not distinguish between highly-reliable and highly-suspicious information or data. It’s just data. That means that as it consumes additional information to continually learn, it isn’t distinguishing between accurate and faulty information.
To make matters worse, AI is learning from AI. We have not yet figured out how to stop AI models from digesting content originally created by AI. This means that AI is bastardizing itself and learning from false or faulty information resulting in compounded inaccuracies with less and less ability to distinguish errors.
The Bias Issue
Much has been made of the inherent biases within AI models. And all the criticism is fair. We see biases in models developing content, resulting in the proliferation of gender and racial stereotypes. For instance, companies using AI to power applicant tracking have run into challenges picking up on key language (think: “executed,”) resulting in more male candidates selected for consideration. There are any number of ways bias finds its way into our work through AI.
Our Response: Evaluate and Reevaluate
All AI models reflect their makers, so we can’t expect them to be able to evaluate and police themselves. In response, we have approached AI in analytics and measurement similarly to all tech development. The paint will remain wet; and the first version will not be the last. We continue to modify and evolve our models to achieve the strongest, most accurate and reliable results possible. We evaluate and reevaluate for bias and errors, consciously building in triggers requiring inspection.
Successfully harnessing this new phase of AI doesn’t eliminate work. It changes it. We spend more time evaluating validity, bias and limitations than we do executing analyses. The ability to edit and critically assess writing is becoming an even more important skill than writing itself. And composing quality prompts as a skill is quickly becoming table stakes.
In the right light, AI is handling the quantity (number of analyses, data sources and more) so that we can focus on quality. As many have noted, AI for AI’s sake isn’t going to get us anywhere special. However, leveraging AI as an enabling tool will create meaningful strides in what we can and will accomplish.
Kate LaVail is Managing Director of Analytics at Ketchum.